Distinctive Features of Deep Learning
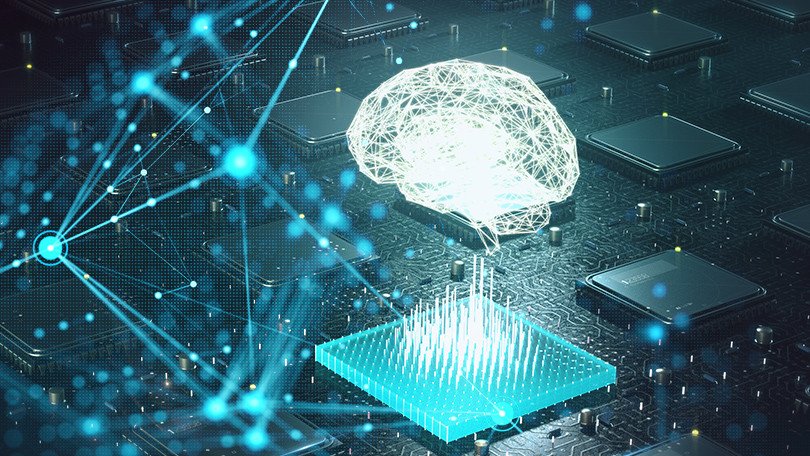
The “Big Data Era” of innovation will give immense measures of chances to new developments in deep learning. Deep Learning is also another area of, Machine Learning research.
Deep learning is a particular type of Machine Learning
To prepare the model, A lot of data is required for an effective deep-learning application. Just as GPU, to quickly process your data. Deep learning is commonly progressively mind-boggling, Two or three thousand pictures, you will require on any occasion to get strong results. here in this article, you know the main difference between Deep Learning and Machine Learning.
As per, the main researcher of China’s significant search engine Baidu, Andrew Ng, and one of the pioneers or leaders of the Google Mind project “The analogy to deep learning is that the rocket engine is the deep learning models and the fuel is the huge amounts of data we can feed to these algorithms.”
In a deep learning framework, each layer of center points plans a specific course of action for features reliant on the past layer’s output. The further you advance into the neural net, the more perplexing the features your center points can see since they aggregate and recombine highlights from the last layer.
A data set can contain dozen to several features. The framework will gain from the pertinence of these features. Be that as it may, not all features are important for the algorithm. An essential piece of AI is to locate a significant arrangement of features to make the framework gets something.
“Deep Learning requires very good quality machines in opposition to conventional Machine Learning algorithms. GPU has turned into a necessary part currently to execute any Deep Learning algorithm. In conventional Machine learning systems, the greater part of the applied features should be distinguished by a space master so as to decrease the unpredictability of the data and make designs increasingly noticeable to learning algorithms to work. The greatest advantage of Deep Learning algorithms as talked about before are that they attempt to take in elevated-level highlights from information in a gradual way. This takes out the need for area mastery and hardcore feature extraction.”
Deep learning models are prepared on huge arrangements of characterized data and neural network models that gain features straightforwardly from the data without the requirement for manual feature extraction. In the event that we draw a diagram or graph indicating how these ideas are based on one another, the diagram is deep, with numerous layers. Therefore, we call this methodology artificial intelligence deep learning “A deep learning framework is an interface, library or a tool which allows us to build deep learning models more easily and quickly, without getting into the details of underlying algorithms. They provide a clear and concise way for defining models using a collection of pre-built and optimized components”
One of the bulk’s well-known sorts of deep neural network systems is known as (Conv Net) convolutional neural systems. A CNN convolves scholarly features with input data, and utilizations 2D convolutional layers, making this design appropriate for preparing 2D data, for example, pictures. CNN wipes out the need for non-automatic component extraction. Distinguish features used to order pictures, so you don’t have. The Conv Net exertion by separating features legitimately(directly) from images. The remarkable features are not pre-trained, they are discovered while the framework plans on a convention of pictures. This self-operating feature derivation makes deep learning representation exceptionally precise for Computer imagination assignments. object characterization.
Key Features of a Keen Deep Learning
- Optimized for execution
- Easy to comprehend and code
- Good circle support
- Parallelize the procedures to diminish calculations
- Automatically process incline
